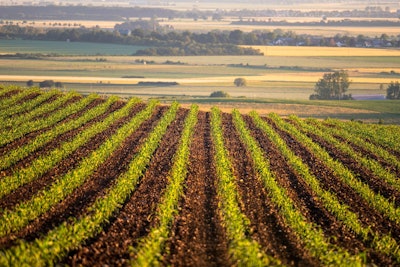
As the world’s population and incomes grow,livestock-related emissionswill increase — unless every step of the supply chain from field to farm commits to implementing sustainable agriculture practices.
While many stakeholders are committed, it’s often difficult for producers to quantify the environmental impact of individual sustainable ag practices, which could mislead climate action and slow the sector’s progress towards net zero.
CarbonSpace calculations inform climate practice decisions
CarbonSpace是一个位于都柏林的启动that set out to take on the challenge of accounting for land-use emissions and sequestration with a satellite-powered platform for carbon footprint tracking.
“Growers and food producers rely on various calculators, which use standard emission factors,” said Lydia Ashburn, growth associate, CarbonSpace.
“As these tools are based on statistics and averages, they can’t provide locally relevant data or detect the quality of practice implementation and its impact in specific conditions.”
The CarbonSpace platform’s proprietary, science-based technology provides emissions estimates unique to individual fields, empowering crop producers to calculate their contribution to reducing the carbon footprint of the overall livestock supply chain.
Many satellite imagery solutions will use a yes/no principle to confirm whether a particular sustainable practice was implemented but still apply standard emission factors to produce a carbon calculation. The CarbonSpace tool, on the other hand, does not rely on any emissions factors or averages.
“At the core of the CarbonSpace technology are machine learning algorithms trained on global multispectral satellite imagery and greenhouse gas (GHG) flux data from ground stations,” Ashburn said.
“As a result, the CarbonSpace tool provides direct estimates on a monthly basis of carbon stock change for each specific field in the supply chain with known accuracy.”
Decades of data provide greater emissions insights
CarbonSpace’s platform accesses satellite and ground station data from as far back as January 2000 to calculate estimates for a field of interest. It then applies its machine learning algorithm to the specific field’s data in any part of the world to prepare estimates for their clients.
“From the start of a new project, we provide over 20 years of historical data on the carbon stock change of the land and follow that with monthly updates,” Ashburn said. “The historical data is available for every month since January 2000, and the updates for each new month come two weeks after the month ends.”
The process is fully remote and automated, requiring no onsite operations.
For producers, it’s a scalable and cost-effective way to estimate the emission and sequestration levels of land and assess the impacts of their sustainable agricultural practices.
Monitoring carbon stock changes in the fields where feed ingredients are grown helps provide greater insights across the entire animal ag supply chain, taking the sector another step closer to net zero.