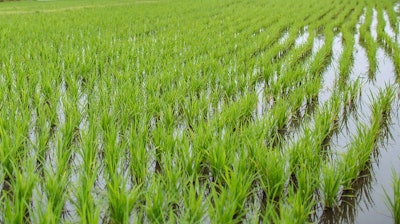
A team of researchers fromOkayama Universityin Japan have developed an artificial intelligence-based app that can estimate the potential yield of rice plants with just a photograph.
By feeding the deep-learning AI system over 20,000 images of rice plants and their corresponding yields, the team created a tool that could revolutionize rice cultivation.
Researchers at Okayama focused on a new technology using recent advancements in AI and machine learning, particularly deep learning with convolutional neural networks (CNNs).
To explore the scope of this new technology, the research team used ground-based digital images taken at harvesting stage of the crop, combined with CNNs, to estimate rice yield. Their study appeared July 28 in Volume 5 ofPlant Phenomics.
The images were captured using digital cameras which could gather the required data from a distance of 0.8 to 0.9 meters, vertically downwards from the rice canopy. With Dr. Kazuki Saito of the International Rice Research Institute (formerly Africa Rice Center) and other collaborators, the team successfully created a database of 4,820 yield data of harvesting plots and 22,067 images, encompassing various rice cultivars, production systems, and crop management practices.
Next, a CNN model was developed to estimate the grain yield for each of the collected images. The team used a visual-occlusion method to visualize the additive effect of different regions in the rice canopy images. It involved masking specific parts of the images and observing how the model's yield estimation changed in response to the masked regions.
The insights gained from this method allowed the researchers to understand how the CNN model interpreted various features in the rice canopy images, influencing its accuracy and its ability to distinguish between yield-contributing components and non-contributing elements in the canopy.
The model performed well, explaining around 68% to 69% of yield variation in the validation and test datasets. Study results highlighted the importance of panicles—loose-branching clusters of flowers — in yield estimation through occlusion-based visualization. The model could predict yield accurately during the ripening stage, recognizing mature panicles, and also detect cultivar and water management differences in yield in the prediction dataset. Its accuracy, however, decreased as image resolution decreased.
Nevertheless, the model proved robust, showing good accuracy at different shooting angles and times of day.
“Overall, the developed CNN model demonstrated promising capabilities in estimating rough grain yield from rice canopy images across diverse environments and cultivars," said Dr. Tanaka. "Another appealing aspect is that it is highly cost effective and does not require labor-intensive crop cuts or complex remote-sensing technologies."
The study emphasized the potential of CNN-based models for monitoring rice productivity at regional scales. The model's accuracy, however, may vary under different conditions, and further research should focus on adapting the model to low-yielding and rainy environments.
The AI-based method has also been made available to farmers and researchers through a simple smartphone application, thus greatly improving accessibility of the technology and its real-life applications. The name of this application is ‘HOJO’, and it is already available on iOS and Android.